Machine Learning and Processing Unstructured Data
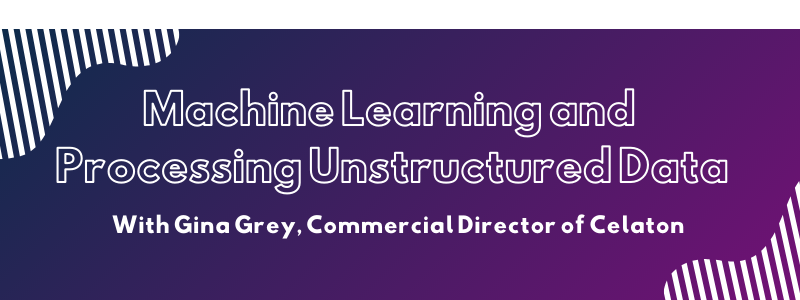
‘Human in the Loop’ Machine Learning and Processing Unstructured Data:
The amount of data organisations receive is on the rise, with the vast majority arriving in the form of documents. These documents are crucial for daily operations and need processing for businesses to gain insight and perform critical actions such as payments or customer responses. It is not always easy for companies to make sense of these documents because of the sheer volume, variety and complexity, particularly as many of the tasks involved with the processing are still manually intensive and repetitive. Crucially, these labour-intensive tasks can result in not only delays but are also error-prone and can lead to low employee morale and negatively impact supplier and customer relationships.
In recent years technology solutions such as Digital Process Automation (DPA), which includes applications such as OCR and RPA, have offered organisations a solution for these manual tasks through automation. DPA is an evolution of Business Process Management (BPM), something organisations have been doing in some form for decades. DPA takes the appropriate manual tasks within a process and utilises computer systems to help organise and perform them more efficiently, eliminating unnecessary repetitive tasks altogether by having the computer system carry them out instead.
Traditional DPA, as defined previously, is extremely effective when structured data is required to be processed because the technology relies on rules-based decision making to perform tasks. For example, DPA could be applied to locate and extract data from a structured spreadsheet and deliver it to a line of business system for further action. In this instance, the location of the data required to be extracted will not change from document to document making it easy for the technology to find the key data with pre-programmed rules. This technology works well when there are high volumes of the same document layout or just a few anticipated variations.
The problem for many organisations, however, is that the data they receive is unpredictable and can be structured, semi-structured or unstructured. Organisations often have very little control over inbound documents and when received at higher volumes can become a real stumbling block for traditional DPA. This is due to the unpredictability of document types making it difficult and ineffective to anticipate and preprogramme the software for every eventuality.
For example, within an Accounts Payable process, an organisation might receive 10,000’s of invoices from a wide variety of suppliers that need processing for payment. Traditional DPA solutions apply a pre-set of business rules to locate, extract and transfer the key data into Finance systems. This solution works effectively when the invoices are structured and follow the same template. However, when an exception is received for example a poor scan, a handwritten note or a new supplier invoice, the preprogrammed rules cannot be applied and a barrier to processing is presented. When an exception occurs, the software would either need to be re-coded or require manual data entry into the Finance system to process, at time and cost to the organisation.
For example, within an Accounts Payable process, an organisation might receive 10,000’s of invoices from a wide variety of suppliers that need processing for payment. Traditional DPA solutions apply a pre-set of business rules to locate, extract and transfer the key data into Finance systems. This solution works effectively when the invoices are structured and follow the same template. However, when an exception is received for example a poor scan, a handwritten note or a new supplier invoice, the preprogrammed rules cannot be applied and a barrier to processing is presented. When an exception occurs, the software would either need to be re-coded or require manual data entry into the Finance system to process, at time and cost to the organisation.
This challenge for document processing is being tackled by the next evolution of BPM, Intelligent Process Automation (IPA). Instead of simply using rules-based decision making as per a DPA application, IPA solutions implement approaches such as Machine Learning, Natural Language Processing and Intelligent OCR to additionally eliminate tasks within a process that normally rely upon a human’s intelligence. In the world of document processing these tasks include; understanding meaning and intent, validation, verification, performing further actions such as customer email responses and handling exceptions. Furthermore, because of the inclusion of technology such as Machine Learning, which is not reliant on structured data and templates, unstructured data and exceptions can be handled within the end-to-end digital process.
Through embracing IPA, document exceptions can be effectively handled because of the essential role that operators perform in a Machine Learning process called ‘Human in the Loop’. In ‘Human in the Loop’, document exceptions are handled within the end-to-end process as there is no requirement to recode or create new templates with every new document received.
For example, Celaton’s IDP platform inSTREAM™ utilises Machine Learning algorithms to learn through the natural consequence of processing and through collaborating with operators who teach it about each document exception. Therefore, as the volume of documents increase, its confidence and accuracy improve to a point where Straight Through Processing (STP) is achieved. As inSTREAM’s confidence and accuracy increases, the amount of interaction needed with humans decreases enabling employees to focus on the complex, value-based tasks. This removes the need for reprogramming and ensures the process is constantly optimised and scalable, saving organisations valuable time, resources and increases productivity in other more valuable areas of the business.
‘Human in the Loop’ is a fundamental next step in the journey of BPM, not only because it enables further automation efficiencies for organisations, but also because it puts people back into processes. For many years, conversations around automation technologies have been dominated by the ‘fear of job losses’ or how to reduce FTEs and this has created a level of mistrust and resistance to adoption. With the rate of data creation, particularly the unstructured type continuing to grow, the collaboration of Machine Learning and employees in systems such as ‘Human in the Loop’ will become essential. Platforms, such as Celaton’s inSTREAM, augment employee’s roles by removing the repetitive manually intensive tasks so that they can complete more rewarding activities such as problem-solving and building relationships. These are activities that are important to an organisation's future success as consumers increase their buying power and markets become more competitive.
In conclusion, whilst organisations are not able to control the types of data they receive, technology advancements such as IPA will enable them to more efficiently process it. Crucially, it is through putting people back into technology and processes that will enable organisations to increase efficiencies and productivity, which will, in turn, support business growth and sustainability in ever demanding and evolving markets.