Improving Service Quality across Shared Services
Add bookmark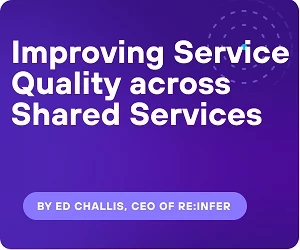
Client service is one of the most competitive and challenging areas of business services. Answering client questions, properly managing expectations and resolving client issues as quickly and efficiently as possible are key to operational efficiency and competitive differentiation for businesses.
But the question is, how do you know you’re doing enough? How do you compare your client service to the competition? And most importantly, how do you know what to improve?
The client service blindspot
Client service in any shared services function is a complex web of different systems and communications channels. It’s difficult enough to maintain visibility over the shared mailbox of a single service desk, never mind managing hundreds or even thousands of mailboxes across an enterprise’s many service teams.
As a result, you have service leaders struggling with poor visibility into client interactions until an event is escalated or a process breaks down completely. They lack the resources to get ahead of problems and proactively manage them. Nor do they have the data to demonstrate where a potential incident was avoided or turned into a positive outcome.
This environment makes the client experience little more than guesswork, and service improvement a process of trial and error. Shared services leaders need the capability to quantify and track a holistic quality of service for the enterprise. Only then can they demonstrate improvements and get ahead of issues before they can snowball into real problems that impact operational or service efficiency.
Measuring quality of service
Key stakeholders lack the visibility and tools needed to quantify, track and improve the quality of service their business provides. They fail to understand client needs, identify and get ahead of issues before they escalate. Furthermore, they struggle to demonstrate real improvements to service levels.
These obstacles have led many shared services leaders to fall back on stakeholder reviews, client interviews and surveys to evaluate their quality of service. Yet these are blunt instruments at best, which can only ever provide a narrow cross-section of opinion. Furthermore, any findings are only useful in hindsight. You won’t be able to pre-empt emerging issues or measure the impact of any changes to your service in real time. It’s akin to driving by only looking in the rear-view mirror.
However, recent advances in natural language processing (NLP) are fuelling massive improvements in how leading shared services centres quantify their quality of service. NLP models are now able to analyse conversations and extract the intent and sentiment of communications between clients and agents, accurately and reliably. Through the model training process, agents are able to impart their subject matter expertise - including their understanding of context and specialist language - giving these models the context they need to interpret situations and client messages accurately.
Through NLP, shared services leaders can understand their client and employee communications at speed and on a massive scale. Machine learning models can then automatically estimate the impact of these communications on the function’s quality of service, aggregating them into a central quality of service score. This opens up the company’s communications - its service - to trend analysis and real-time monitoring.
Automated alerts can be set up that are triggered by specific events or deviations from acceptable quality of service levels. Service issues can be identified and resolved before they even have the chance to impact operational efficiency. It paves the way for a client service that is real-time, responsive, constantly monitored and continuously improving.
Achieving continuous service improvement
To measure and monitor their quality of service, service leaders need to be able to extend analysis across all client messages and communications channels. Manual, analogue methods fail to achieve the coverage and responsiveness required.
That’s why Re:infer has launched Quality of Service, a new capability that uses powerful NLP and machine learning to capture context-specific client intents. Quality of Service provides a new capability for service functions to accurately track and improve their service, with real-time monitoring and customised alerts across all touchpoints and service teams.
Quality of Service uses in-depth sentiment, emotion and business-specific intent analysis to help service leaders understand the quality of service their business delivers and get ahead of operational issues - in real time and at scale.